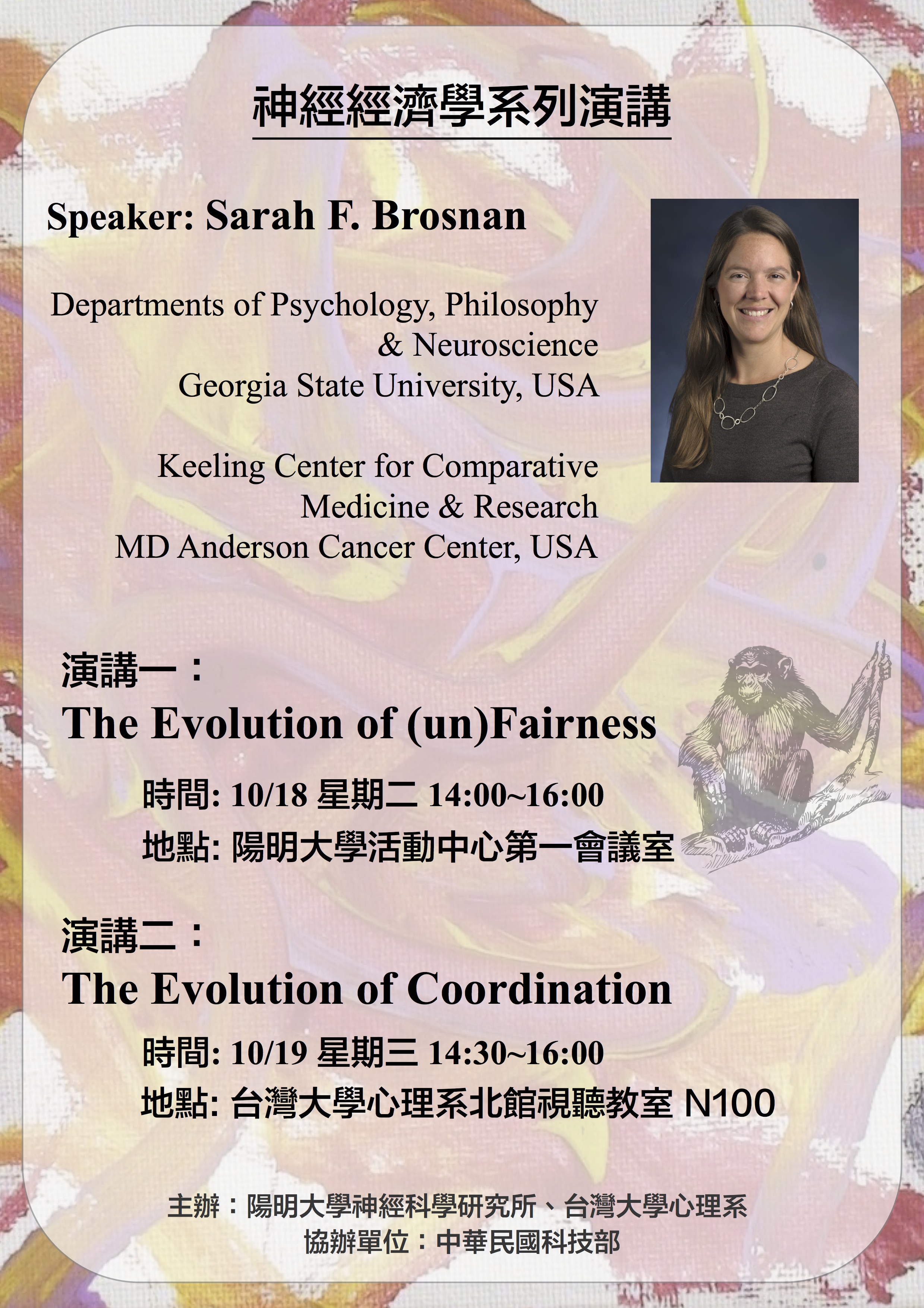 |
|
Sarah Brosnan
Departments of Psychology, Philosophy & Neuroscience Georgia State University, USA Keeling Center for Comparative Medicine & Research MD Anderson Cancer Center, USA
The Evolution of (un)Fairness
The human sense of fairness is an evolutionary puzzle; why do we put so much value on what we receive relative to others? One answer to this question emerges from studying other species. Although fairness itself is empirically in- tractable, it can be translated empirically into responses to reward distribu- tion, and studying other species’ reactions can tell us something about the evo- lution of our own behavior. Indeed, humans are not alone in disliking inequity in reward distributions; many species protest receiving less than a partner for the same task, and this tendency occurs primarily in species that cooperate outside kinship and mating bonds, indicating a link between the two behaviors. However, a full sense of fairness requires not only this, but also that individuals notice and seek to equalize outcomes to their own detriment. There is less evi- dence of this latter reaction nonhuman species, although it has been docu- mented in our closest relatives, the apes. This reaction probably reflects an at- tempt to forestall partner dissatisfaction with obtained outcomes and its nega- tive impact on future cooperation. Therefore, it is likely that the evolution of this response, combined with advanced abilities at inhibition and planning, al- lowed the development of a complete sense of fairness in humans, which func- tions not to provide equality for its own sake, but for the sake of continued suc- cess in cooperation.
|
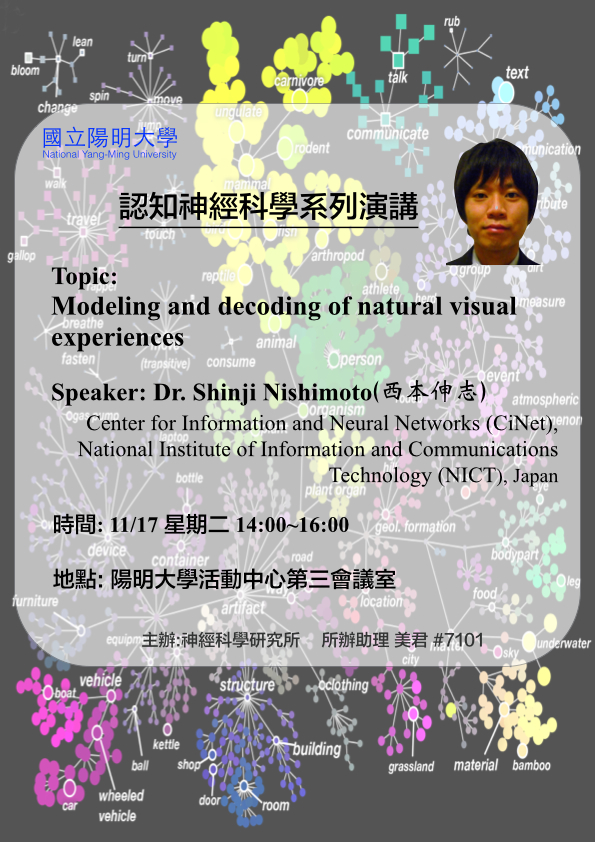 |
|
Shinji Nishimoto
Center for Information and Neural Networks, National Institute of Information and Communications Technology, Japan
Modeling and Decoding of Natural Visual Experiences
One of the goals of studying systems neuroscience is to understand how the brain works under day-to-day, natural conditions. Pursuing such a goal inevitably involves the use of two kinds of high-dimensional data: one of them is dynamic spatiotemporal patterns of perceptual and cognitive experience. The other is spatiotemporal patterns of brain activity that represent those internal experiences. We aim to understand the brain by building predictive models that explain the relationship between these two kinds of high-dimensional patterns. Such models reveal quantitative representations inside the brain, such as space-time visual representations in early visual areas, semantic representations in occipitotemporal areas, and their dynamic warps during cognitive tasks. We have also built brain decoders that can quantify and visualize perceptual experiences from brain activity in humans. As a whole, modeling of the brain provides a general and quantitative foundation for understanding human perception and cognition.
|
|
References: Çukur, Nishimoto, Huth, Gallant. Nature Neuroscience 16(6):763-70. (2013)
Huth, Nishimoto, Vu, Gallant. Neuron 76(6):1210-24. (2012)
Nishimoto, Gallant. The Journal of Neuroscience 31(41):14551-64. (2011)
Nishimoto et al., Current Biology 21(19):1641-6. (2011) |
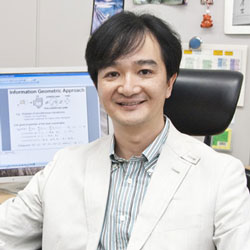 |
|
Hiroyuki Nakahara
Laboratory for Integrated Theoretical Neuroscience, RIKEN Brain Science Institute, Japan
Neural Computations for Social Decision-Making: Learning to Simulate Others’ Decisions
A fundamental challenge in social cognition is elucidating how one learns and predicts the mind of others, and what the underlying neural mechanisms are. We approach this challenge by using computational frameworks to link behavior and neural systems. Extending reinforcement learning theory into the realm of social cognition and combining human fMRI with modeling, we recently addressed how one learns to simulate the decision-making of others. We found that learning is realized by using two simulation signals in a hierarchical arrangement, pointing to a basic mechanism for learning others’ minds. We discuss about new avenues of inquiry brought by identifying key computational primitives in neural processes that constitute apparently complex social decision making.
|
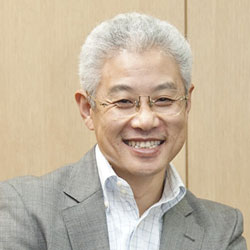 |
|
Kang Cheng
RIKEN Brain Science Institute, Japan
High-Resolution fMRI Studies of Human Visual Cortex
In this talk, I will first briefly review our previous efforts exploring human primary visual cortex (V1) with high-resolution (sub-millimeter resolution) functional magnetic resonance imaging (fMRI) [1-5], and then present the results from three unpublished studies, all conducted on a 4 Tesla MRI system. In the first study, using both direct mapping and indirect decoding approaches, we demonstrated that orientation selectivity in human V1 can be relaibly revealed. In particular, we found that large dining veins contribute significantly to orientation-selective BOLD signals. These results are strikingly surprising and invite more detailed anatomical and functional studies of draining veins at various spatial scales. In the second study, we manipulated the spatial resolution of fMRI data and analysed the geometric relationship between 1) the spatial resolution of imaging voxels, 2) the systematic increase and decrease in the proportion of direction-selective voxels, and 3) the repetition periodicity of assumed underlying direction-selective hypercolumns in the human middle temporal complex (hMT+). Using this approach, we were able to indirectly estimate the average size of hypercolumns for motion direction in hMT+, which was approximately 0.7 mm in the narrow dimension. Finally, in the third study elucidating the object-selective responses in human occipito-temrpoal cortex, I will highlight the unique advantage of doing these experiments at higher resolutions.
|
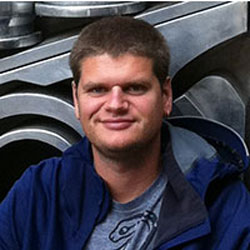 |
|
Ian Krajbich
Assistant Professor in the Department of Psychology and Department of Economics, Ohio State University
A Common Mechanism Underlying Value-based Decision Making
People make numerous decisions every day including perceptual decisions like walking through a crowd, decisions over primary rewards like what to eat, and social decisions that require balancing own and others’ benefits. The unifying principles behind choices in various domains are, however, still not well understood. Mathematical models that describe choice behavior in specific contexts have provided important insights into the computations that may underlie decision making in the brain. However, a critical and largely unanswered question is whether these models generalize from one choice context to another. Here I show that a model adapted from the perceptual decision-making domain and estimated on choices over food rewards accurately predicts choices and reaction times in three independent sets of subjects making social decisions. The robustness of the model across domains provides strong evidence for a common decision-making process in perceptual, primary reward, and social decision making. I also provide evidence that visual attention is a critical variable in this process.
Benefits of Neuroeconomic Modeling: New Policy Interventions and Predictors of Preference
From its inception, neuroeconomics has promised to use knowledge from neuroscience and psychology to improve models of economic decision making. Here we deliver on this promise by introducing a biologically-plausible (drift-diffusion) model of decision making that is able to predict behavior, with fixed parameters, in random groups of individuals across very different tasks. The model also goes beyond the traditional economic approach because it is able to predict not only choices but reaction times as well. But why should economists care about modeling reaction times? For one, as predicted by the model, we consistently observe that people spend too much time on irrelevant choices for which they are nearly indifferent. In a world of time constraints, time spent on unimportant decisions represents an opportunity cost, namely time not spent on more important decisions. To help subjects overcome this inefficient allocation of time, we designed an intervention that puts a time limit on subjects’ choices. This simple intervention substantially improved subjects’ welfare in a time-constrained choice experiment. Second, the precise relationship between reaction times and choice probabilities implies that reaction times can be used to predict indifference points and the strength of preferences. We demonstrate this ability using data from an Ultimatum Game experiment. Overall, we show that better understanding the process by which people make decisions can explain the basis for stochastic choice behavior and help us do both normative and positive economics.
|
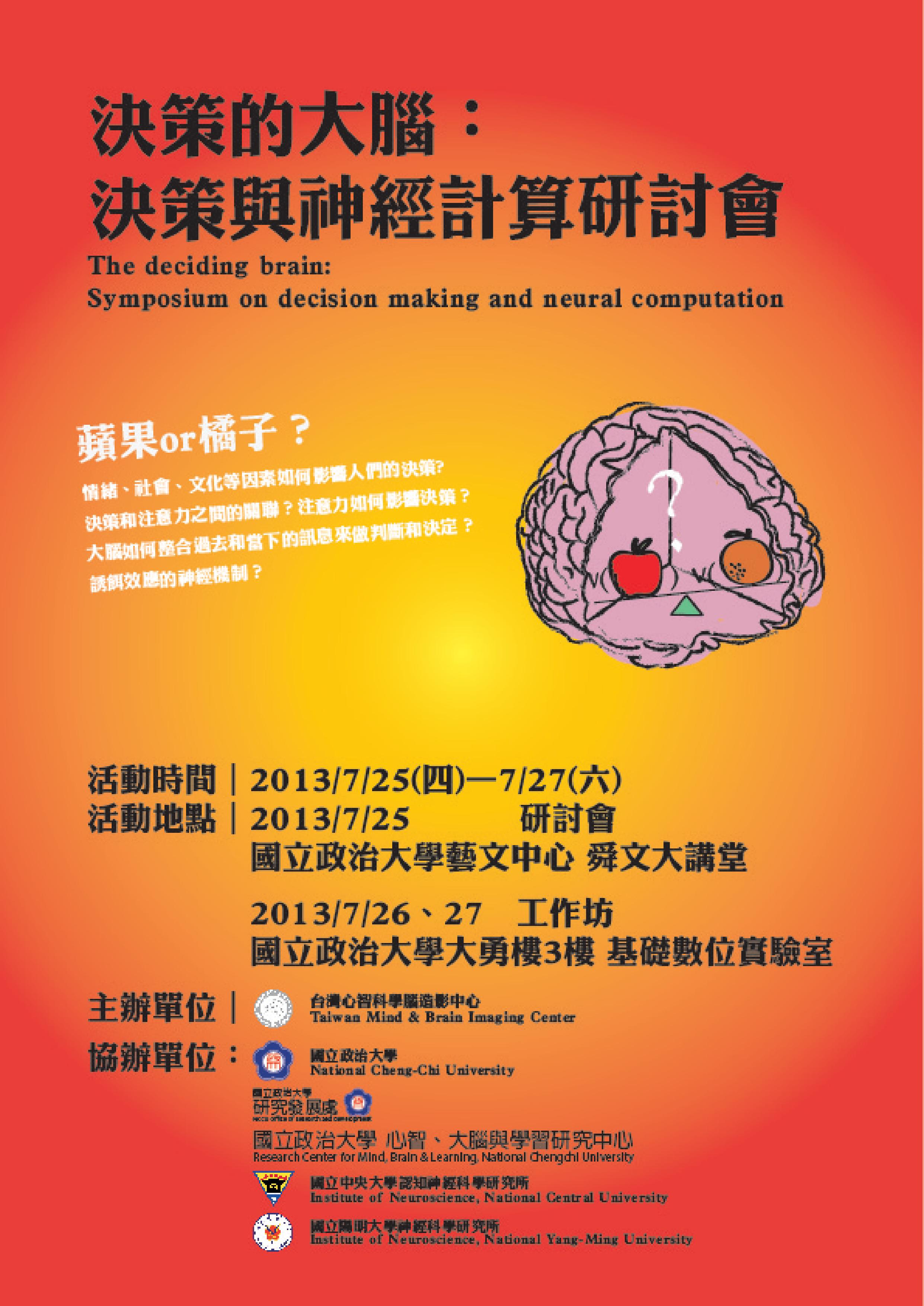 |
|
The deciding brain: Symposium on decision making and neural computation [program book]
|
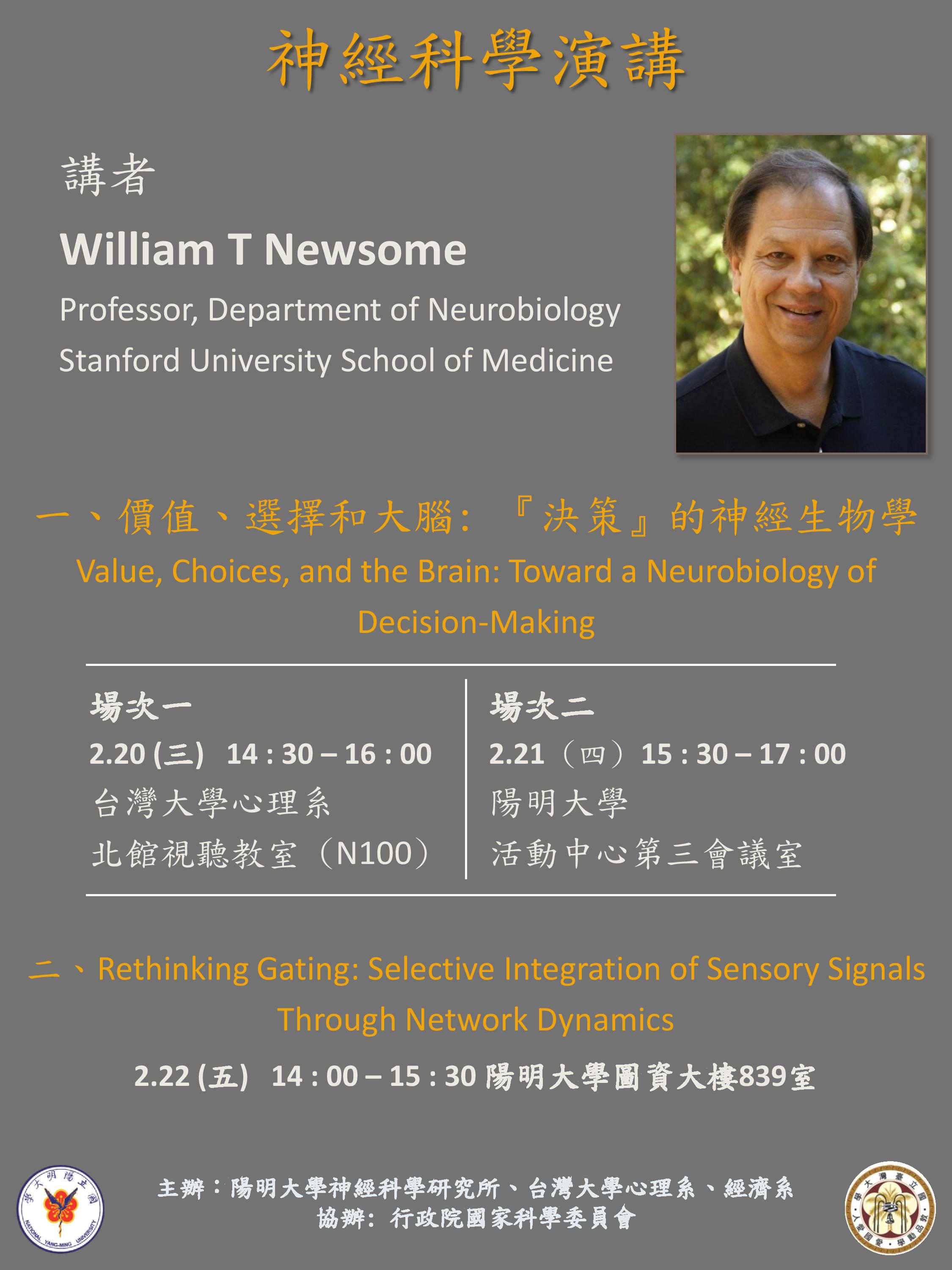 |
|
William Newsome
Professor, Department of Neurobiology, Stanford University School of Medicine
1 & 2. Value, Choices and the Brain: Toward a Neurobiology of Decision-Making
Decision-making is a critical cognitive capacity that links perception to action. Sensory physiologists emphasize the effects of sensory inputs on our decisions, but psychologists and economists have long known that decisions are also strongly influenced by prior experience concerning the “value” of alternative choices, expressed in terms of likely positive or aversive consequences. Thus, brain circuitry that mediates decision-making should reflect both sensory and value influences. We have recently been able to demonstrate both effects in neurophysiological recordings from the frontal and parietal lobes of awake, behaving animals. I will review these empirical data and consider their implications for understanding how the brain makes decisions.
3. Rethinking Gating: Selective Integration of Sensory Signals Through Network Dynamics
A hallmark of decision-making in primates is contextual sensitivity: a given stimulus can lead to different decisions depending on the context in which it is presented. This kind of flexible decision-making depends critically upon gating and integration of context-appropriate information sources within the brain. We have analyzed neural mechanisms underlying gating and integration in animals trained to perform a context-sensitive decision task. Surprisingly, both relevant and irrelevant sensory signals are present within frontal lobe circuits that form decisions, implying that gating occurs very late in the process. Dynamical systems analysis of the neural data, combined with a dynamical recurrent network model, suggests a novel mechanism in which gating and integration are combined in a single dynamical process.
|
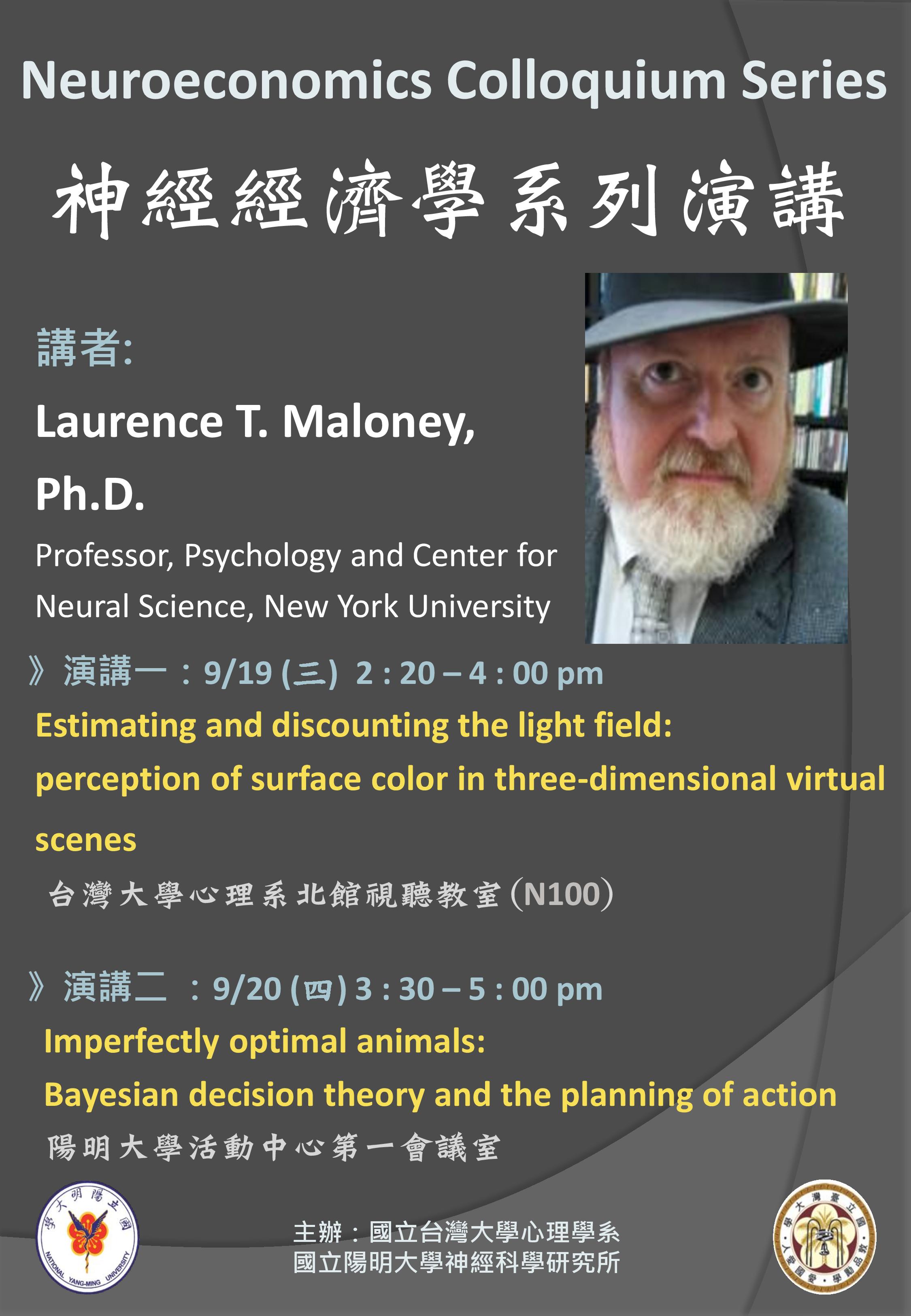 |
|
Laurence T. Maloney
Professor, Psychology and Center for Neural Science, New York University
1. Estimating and discounting the light field: perception of surface color in three-dimensional virtual scenes
In everyday scenes, the intensity and chromaticity of the light absorbed by a matte surface depends on its location and orientation. I will first describe recent experiments intended to investigate surface color and lightness perception in 3D rendered scenes. We found that the visual system partially compensates for changes in illumination due to changes in location and orientation of test surfaces. We show that, in carrying out these experimental tasks, observers effectively represent the spatial distribution, chromaticities and relative intensities of light sources in the scene. I’ll describe additional experiments where we assess how the visual system estimates the distribution of light in scenes (the light field) and how it used this information in estimating surface colors.
2. Imperfectly optimal animals: Bayesian decision theory and the planning of action
The movement we plan is not always the movement we execute. Any discrepancy is the consequence of own intrinsic motor uncertainty. I will first describe a model of movement planning based on Bayesian decision theory that takes our own visual and motor uncertainty into account in selecting movement strategies and present experimental evidence suggesting that human movement planners deviate slightly but systematically from optimal in many tasks (Schüür et al, under review; Wu et al, 2009, 2011; Zhang et al, under review; Zhang & Maloney, 2012). While human performance is impressive in all of the tasks considered, it is not optimal, and deviations from optimality are potentially a valuable source of information concerning how humans represent and make use of information about uncertainty (Zhang & Maloney, 2012).
|
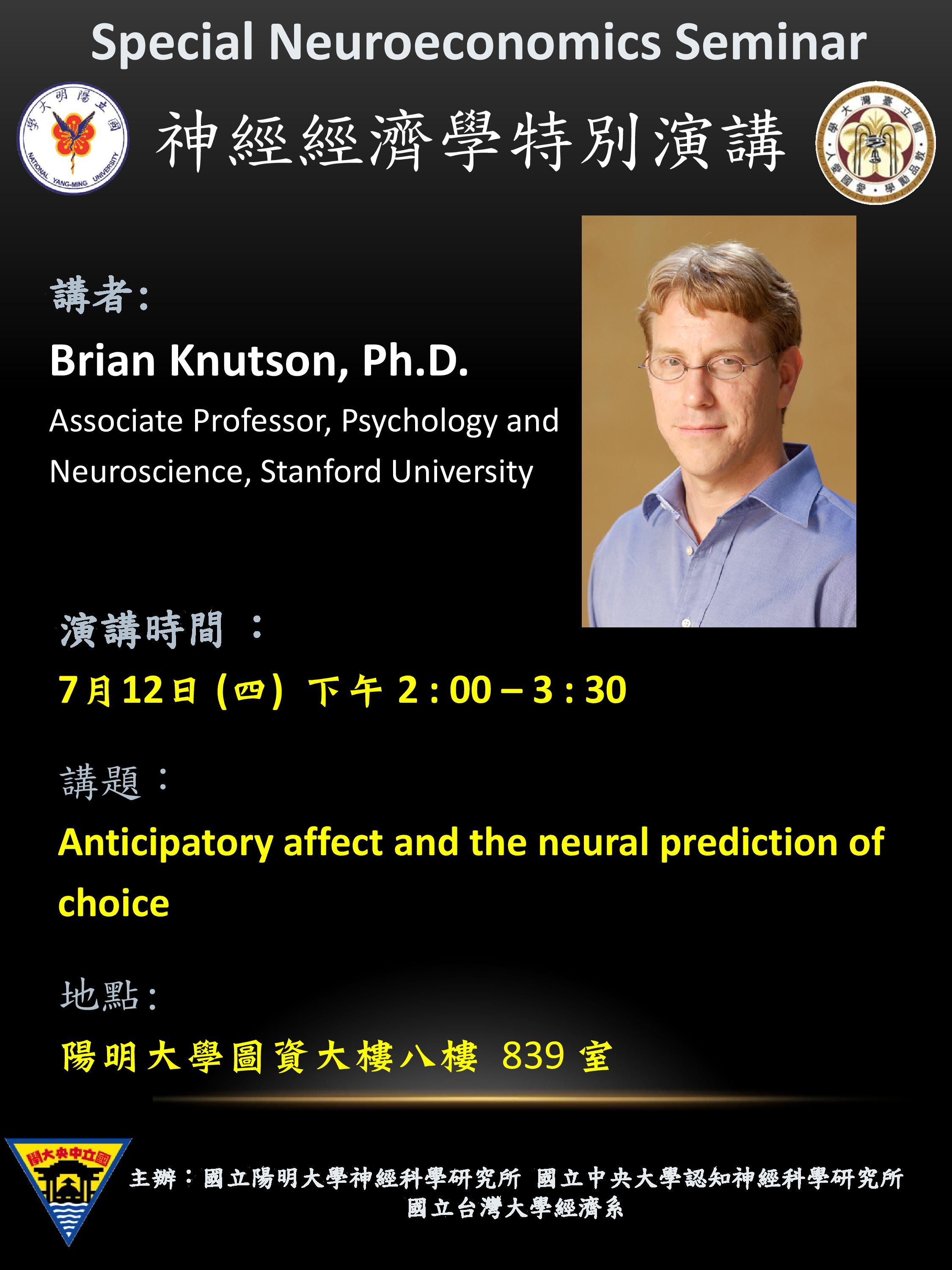 |
|
Brian Knutson
Associate Professor, Psychology and Neuroscience, Stanford University
Anticipatory Affect and the Neural Prediction of Choice
Affect occurs not only in response to choice outcomes, but also can precede and influence choice. The spatial and temporal resolution of functional magnetic resonance imaging (fMRI) now allows investigators to visualize changes in neural activity seconds prior to choice. I will review efforts in our laboratory to use fMRI to visualize neural correlates of "anticipatory affect," and then to use that activity to predict upcoming choice in domains ranging from investing to shopping. While current findings suggest that neural activity related to anticipatory affect can be used to predict choice, future optimizations of design and analyses promise to improve these predictions.
|
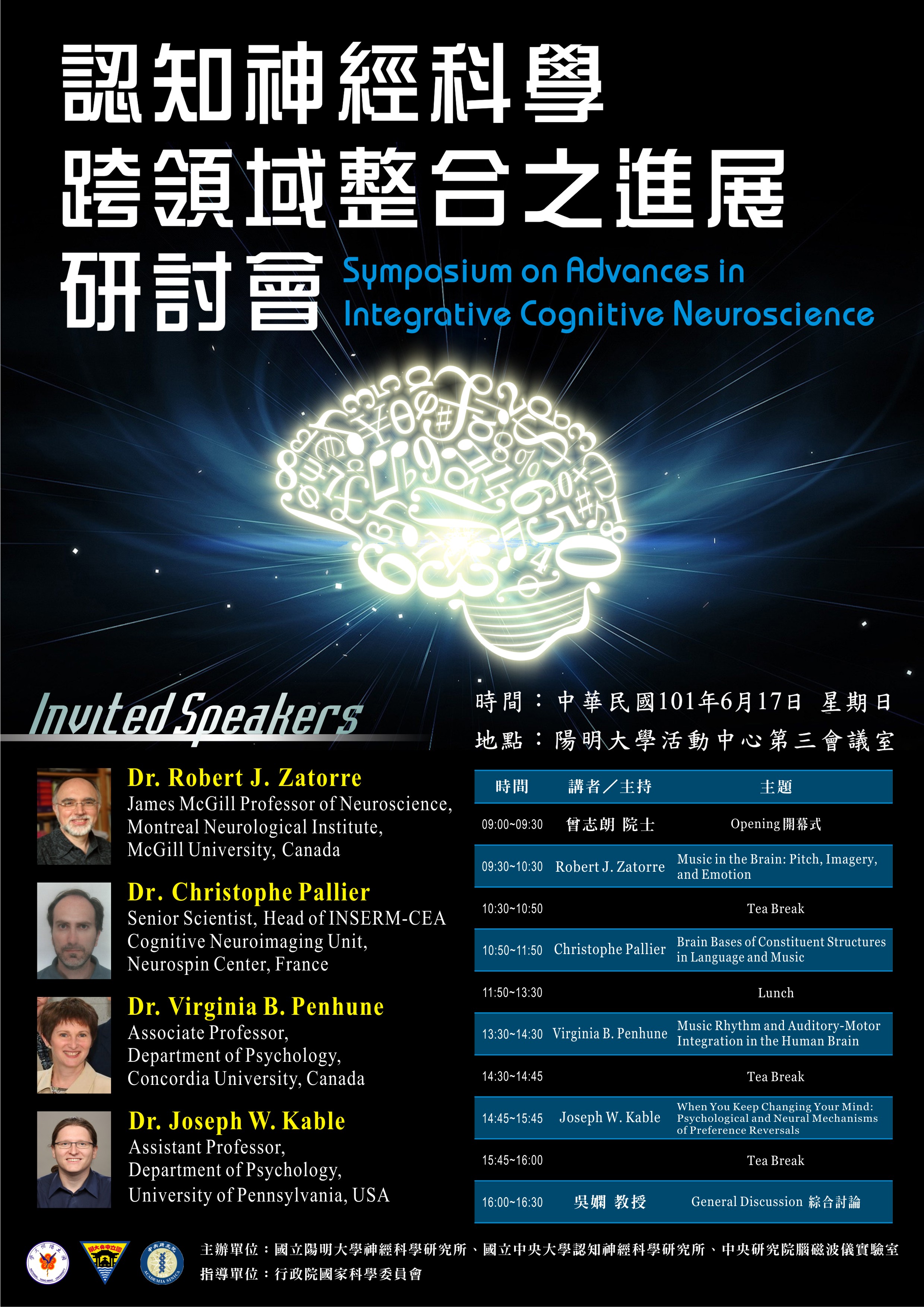 |
|
Joseph Kable
Psychology Department at University of Pennsylvania
1. Sustaining Delay of Gratification: Potential Cognitive and Neural Mechanisms
Persistence in the pursuit of long-run outcomes is an important dimension of self-control. Decision-makers often fail to persist: they initially choose a larger, later outcome, wait a period of time, and then abandon this initial choice in favor of a smaller, immediate reward that had always been available. Explanations for this kind of dynamic reversal usually assume that the timing of outcomes is known with certainty, but this is rarely the case in the real world. When outcome timing is uncertain, a rational decision-maker should continually update their estimate of the remaining delay. The value of continued persistence therefore hinges critically on the decision-maker's prior expectations regarding the temporal uncertainty. We first show that, with respect to several self-control dilemmas, decision-makers hold temporal expectations that would rationalize persistence failure at some point. In a series of behavioral experiments, we then demonstrate that people adapt their degree of persistence depending on the statistics of the environment, waiting longer for delayed rewards when persistence is profitable, and exhibiting a reduced willingness to wait when reversals are merited. Finally, we show that when individuals wait for delayed rewards, BOLD activity in medial prefrontal regions reflects a continually updated estimate of the delayed reward's value. This activity varies depending on the timing statistics of the environment, and predicts whether an individual will abandon the delayed outcome or continue to persist. These results demonstrate the critical role of temporal expectations in persistence, and suggest that shaping these expectations could encourage persistence when this is desired.
2. When you keep changing your mind: Psychological and neural mechanisms
of preference reversals
Systematic inconsistencies in people's decisions provide a central challenge to rational choice theories. A classic example is the "preference reversal phenomenon": for two gambles matched in expected value, people systematically choose the higher-probability option but provide a higher bid for the option that offers the greater amount to win. Here we use eye-tracking and functional brain imaging to help better understand the mechanism underlying such reversals. We find that the preference reversal phenomenon is accompanied by a shift in visual attention to different attributes, with people fixating probabilities more during choices and payoffs more during bids. We also find a corresponding change in the influence that different attributes have on neural signals linked to the computation and comparison of subjective values. These findings support a "contingent weighting" explanation of preference reversals, which locates the source of the reversal in a task-dependent change in the weight given different attributes in the valuation process.
|
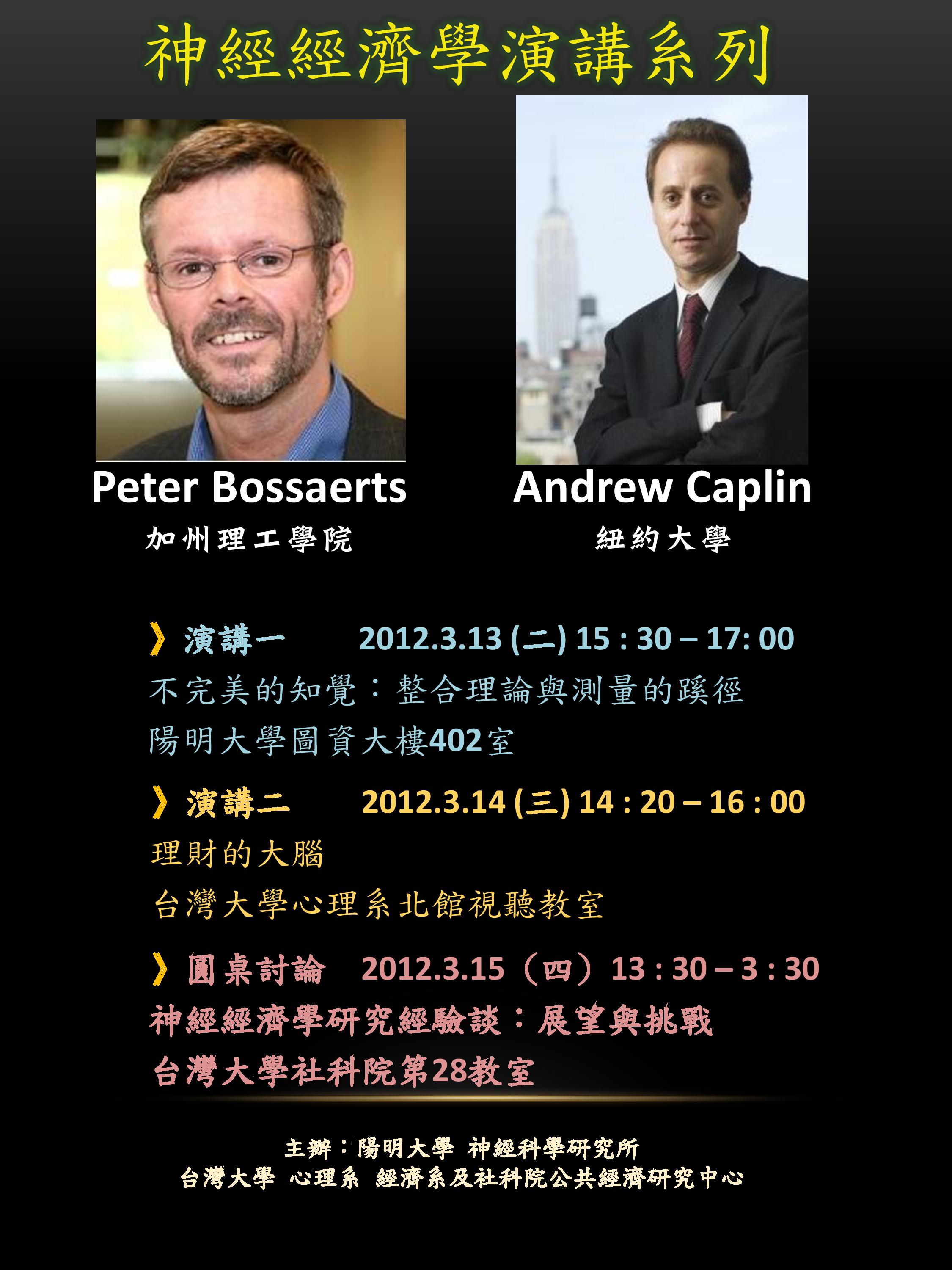 |
|
Andrew Caplin
Silver Professor of Economics, New York University
Co-director, NYU Center for Experimental Social Science
Peter Bossaerts
William D. Hacker Professor of Economics and Management
Professor of Finance, California Institute of Technology
1. Imperfect Perception: An Integrated Approach to Theory and Measurement (Prof. Andrew Caplin)
Psychologists and economists share an interest in how perceptual constraints impact choice behavior. The talk outlines a new approach to this subject in which theory and experiment are tightly integrated. The results to date provide a proof in principle that this new approach produces valid insights into the link between attentional effort and decision making mistakes. The potential for richer measurement to enhance our understanding of this linkage is stressed.
2. The Human Brain Behind Financial Skill (Prof. Peter Bossaerts)
The talk explores the neurobiological foundations of human decision making under uncertainty. What is the role of emotions? How does the brain value uncertain prospects? How exactly do important neuromodulators such as dopamine and norepinephrine influence assessment of risky outcomes? Are social risks encoded differentially, or even separately? What algorithms does the brain use to learn risks through experience? Is problem complexity the right way to define the limits of human rationality?
3. Dialogues on Doing Research on Neuroeconomics: Values and Challenges   (Roundtable discussion with Prof. Andrew Caplin and Prof. Peter Bossaerts)
In this roundtable discussion, Dr. Caplin and Dr. Bossaerts will share with us their experience in doing research on neuroeconomics and in collaborating with neuroscientists. In particular, they will discuss, from the perspective of someone whose original training was in economics or finance, the value of meeting neuroscience and psychology and the challenges neuroeconomics as an interdisciplinary field faces. Researchers and students from all areas of research are welcome to attend. We especially encourage students with economics background to join us on this roundtable discussion.
|
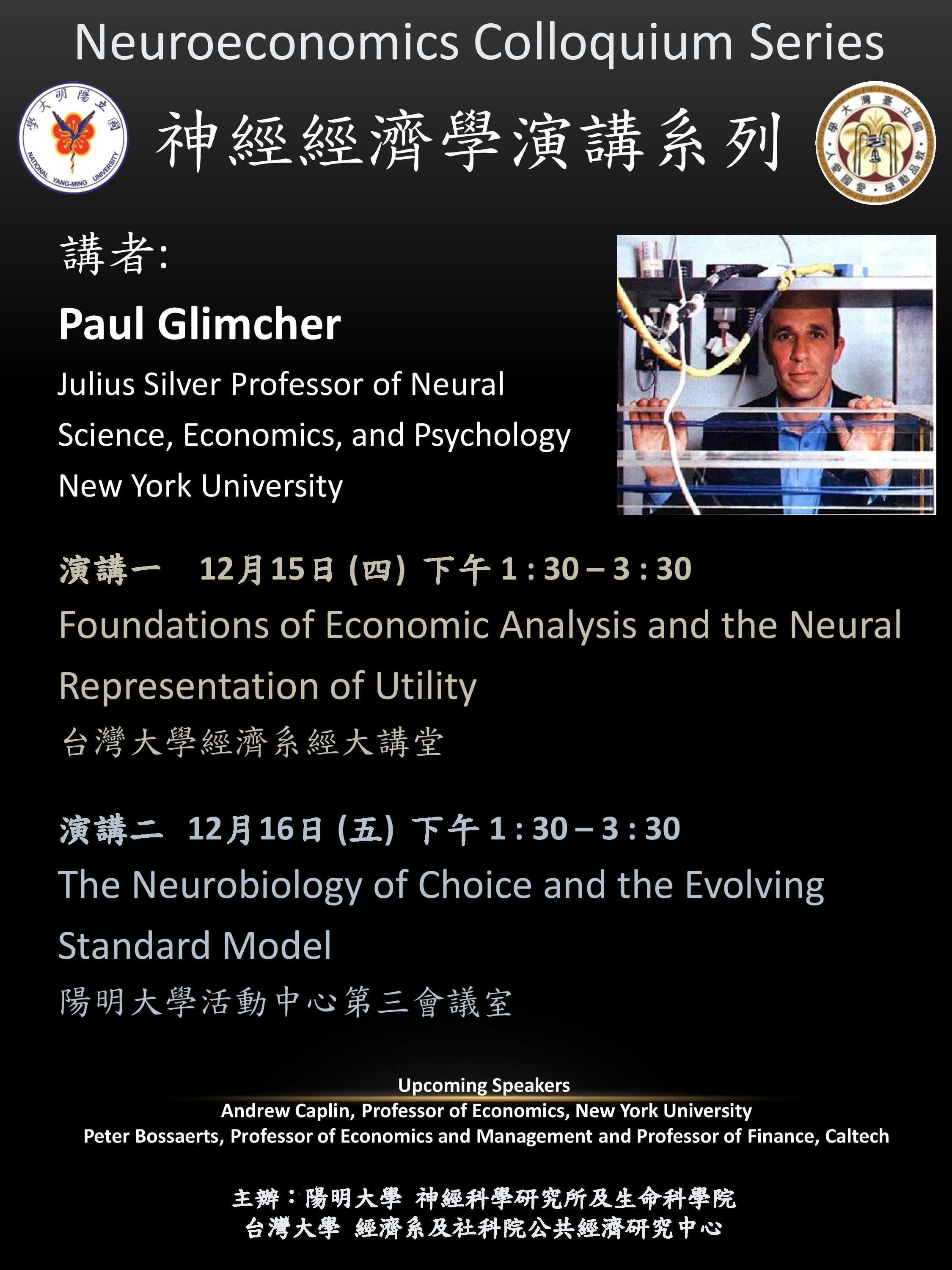 |
|
Paul Glimcher
Julius Silver Professor of Neural Science, Economics, and Psychology
New York University
1. Foundations of Economic Analysis and the Neural Representation of Utility
Over the past decade there has been a significant debate about how economics and neuroscience will influence each other. In this presentation I will argue that a meaningful synthesis of these disciplines will emerge only when serious economic theory is used to guide neurobiological inquiry. If this occurs I argue that it will yield a new class of economic theories that make both economic and neurobiological predictions, both of which can be meaningfully tested. To that end I will describe an axiomatic analysis of the neural representation of utility shocks. Based on fMRI data I will argue for the existence of a utility-like representation in a specific brain area. I will then show that a set of neural measurements in this brain area can predict later choice data. Differences between economic utility and the cardinal object we refer to as "subjective value", and the implications of these findings for cardinal theories of utility will be discussed.
2. The Neurobiology of Choice and the Evolving Standard Model
There is now broad agreement in the neurobiological community about the basic features of the neurobiological mechanism for human and animal decision making. I will review this emerging standard model and then present two specific studies. They will show how economic theory can inform neurobiological studies of brain mechanisms in a highly valuable way - modifying existing neurobiological theory. The second will show the reverse: neurobiological data that makes fundamentally novel predictions about inconsistent human choice behavior.
|
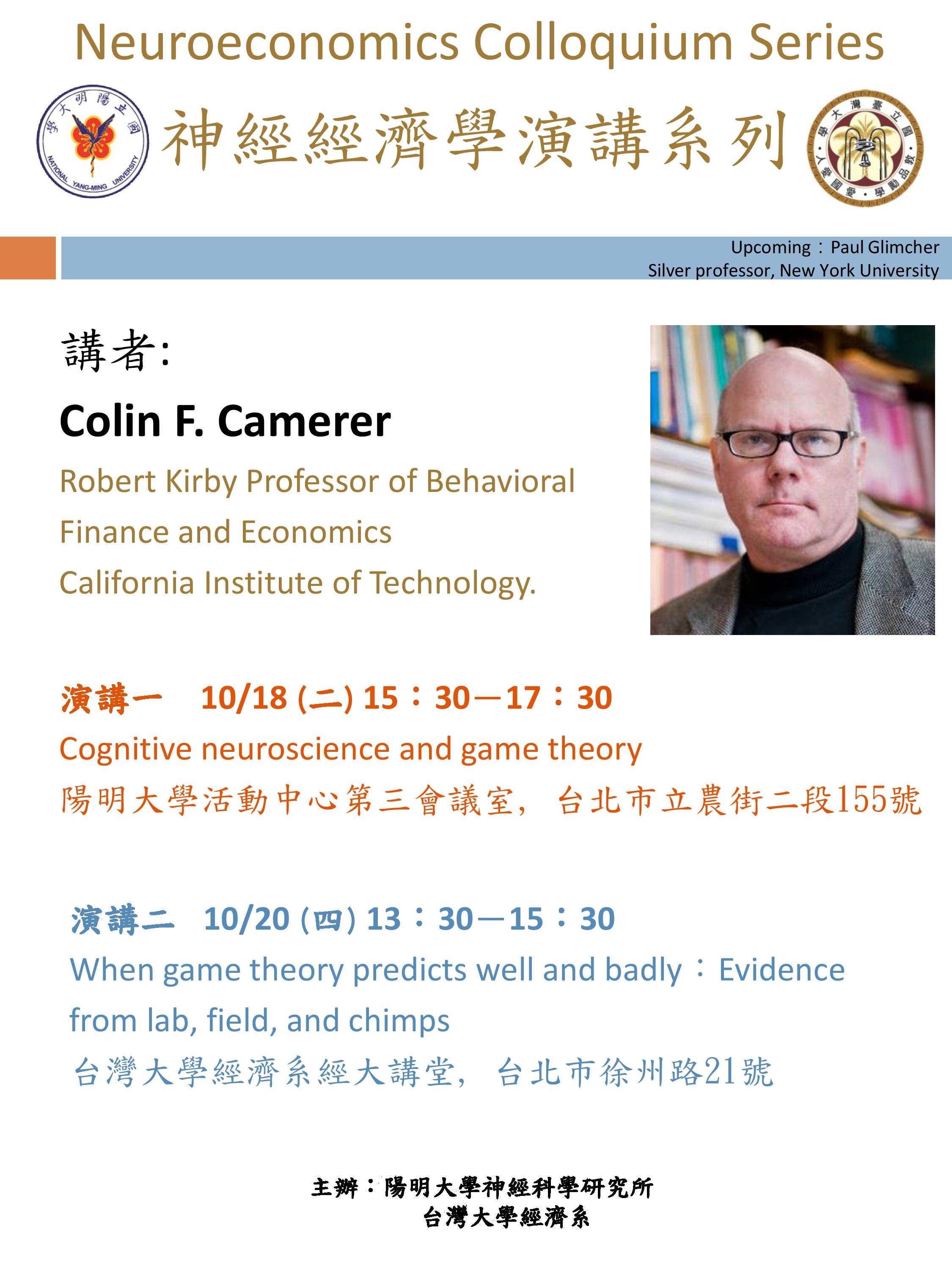 |
|
Colin F. Camerer
Robert Kirby Professor of Behavioral, Finance and Economics, California Institute of Technology
1. Cognitive neuroscience and game theory
Game theory provides a vocabulary of canonical strategic interactions that are important in human social, political, and economic life. However, game theory has not been applied in cognitive neuroscience very much. This talk will discuss some recent studies using fMRI and disorders (autism) to illustrate how game theory might be useful in cognitive neuroscience.
2. When Game Theory Predicts Well and Badly:
Evidence from Lab, Field, and Chimps
There is mixed evidence of how accurate game theoretic predictions are compared to human behavior. Cognitive hierarchy models can reconcile this evidence systematically, since they sometimes predict large deviations and sometimes predict small deviations. This will be illustrated with lab behavior, eyetracking, and field data from a Swedish lottery. An unusual domain in which game theory may work surprisingly well is when a particular species has evolved a special niche-dependent adaptation for certain types of fitness-enhancing games. This is addressed by comparing humans and chimps.
|
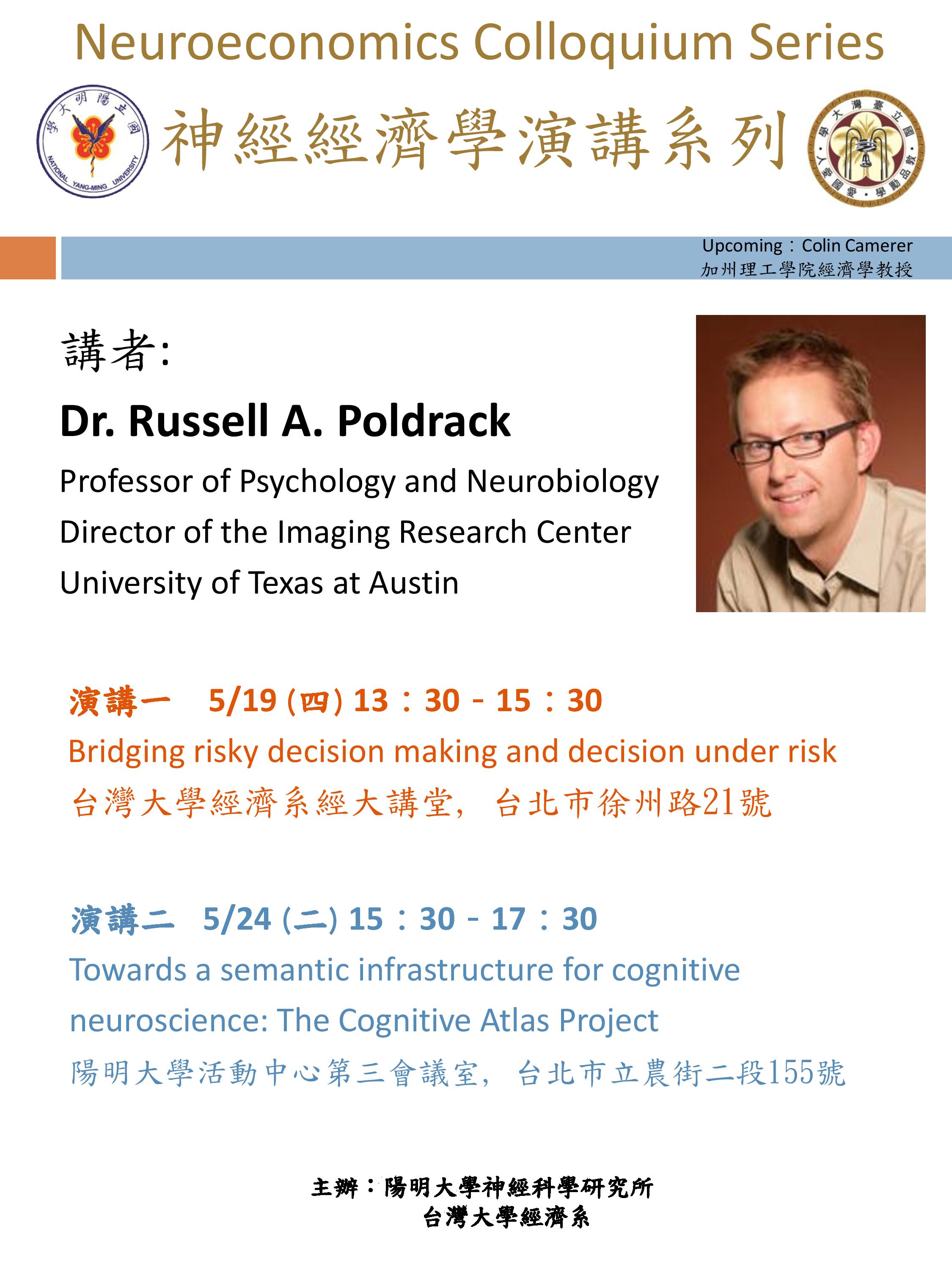 |
|
Russell Poldrack
Professor of Psychology and Neurobiology, Director of the Imaging Research Center
University of Texas at Austin
1. Bridging Risky Decision Making and Decision Under Risk
Neuroeconomics has provided substantial insights into the processes underlying economic concept of "decision under risk." However, this work has largely failed to provide insights into "risky decision making", a lay concept with significant public health implications. I will discuss the relation between these concepts, and outline how dynamic decision making tasks provide the potential to bridge the gap between these two concepts.
2. Towards a Semantic Infrastructure for Cognitive Neuroscience: The Cognitive Atlas Project
We are drowning in results from neuroimaging studies, but starving for an understanding of how these results inform brain function. In order to integrate across this wealth of research findings, I propose that we need a formal semantic infrastructure similar to those developed in other areas of bioscience such as genomics. I will first describe a set of informatics tools that mine literature and neuroimaging databases to characterize the relation between neural and mental function. I will then describe the Cognitive Atlas project (www.cognitiveatlas.org), which aims to develop a knowledge base for mental structure. Finally, I will outline a proof of concept showing how such a database could be used to extract the latent structure of brain processes that underlie mental function.
|
|
|